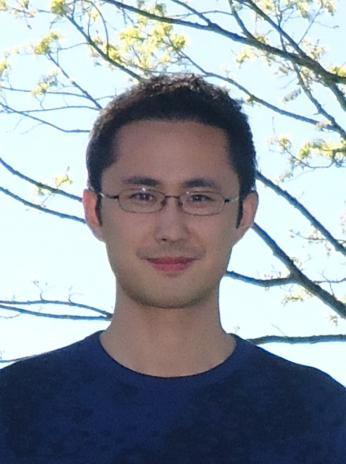
Topic: Reinforcement-learning-based control of convectively-unstable flows
Time: August 3rd 15:00pm, Wednesday
Location: Room 3#307
Speaker: Prof. Mengqi Zhang
Inviter: Prof. Ryohei Seto
Abstract: Flow control is an important interdisciplinary topic in fluid mechanics. Based on the control theory, it can deal with optimisation problems in fluids, such as reducing the resistance in a flow or maximizing the mixing efficiency. This talk reports our recent work that uses a machine learning method to study flow control problems in convectively unstable flows More specifically, we apply the deep reinforcement learning (DRL) method to suppress perturbations in the 1-D linearized Kuramoto-Sivashinsky (KS) equation and in the 2-D boundary layer flows over a flat plate. We found that the DRL strategy is robust to both measurement and external noise. With the gradient-free Particle Swarm Optimization algorithm, we also determined the best strategy for the sensor placement. This work demonstrates for the first time the ability of the reinforcement learning method to control the convectively unstable flows.